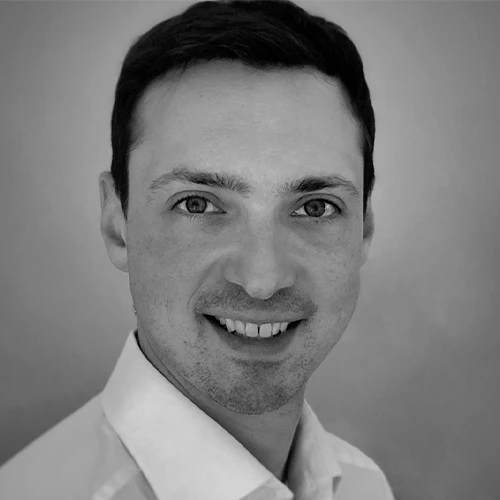
Expert Spotlight
Lorenz Rognoni, PhD
Portfolio Owner, Spatial Image Analysis
“I really like how Ultivue has everything, end-to-end, in one organization. We start with determining the best possible assay for the customer, collect the data using an InSituPlex assay, and then apply the latest deep learning models to the analysis.”
Background
Lorenz Rognoni, Spatial Image Analysis Portfolio Owner at Ultivue, wasn’t always working in computational pathology. His first interest was physics, and he pivoted to biophysics during his graduate work to apply quantitative methods to biology. He wanted to be closer to technologies that can be applied to patients, so that led him to computational pathology.
Before joining Ultivue, he optimized quantification methods for both brightfield and immunofluorescence multiplex IHC assays at Definiens and later at AstraZeneca. With years of experience in the field, Lorenz possesses a deep understanding of the challenges associated with image analysis.
Role at Ultivue
At Ultivue, Lorenz has ample opportunity to use advanced image and data analysis technologies to help customers interpret the spatial information available within each clinical research sample.
Lorenz and his team have developed their own highly optimized, up-to-date, and scalable cloud-based image analysis pipeline. Images, primarily generated through Ultivue’s Assay Services, are analyzed to quantify biomarkers within tissues, identify cell types, and define sample phenotypes, providing a better understanding of which cells and proteins are involved in disease.
For high-throughput applications, results from this analysis pipeline have been above and beyond what a third-party software can achieve.
Looking ahead
Lorenz wants immunofluorescence (IF) to be a primary endpoint in the clinic. He believes that the biggest issue facing some multiplex IF methods is signal variability, which is why brightfield IHC continues to be the primary method for clinical pathology. Lorenz and his team seek to change that paradigm by leveraging the power of AI to mitigate the challenges associated with immunofluorescence.
Rather than focusing solely on signal intensity thresholds, Lorenz and his Image Data Science team train Deep Learning models to define positive and negative cellular phenotypes based on morphology and other important contextual details. This enables Ultivue’s analysis methods to accommodate variability in signal intensity and deliver robust, reproducible data.
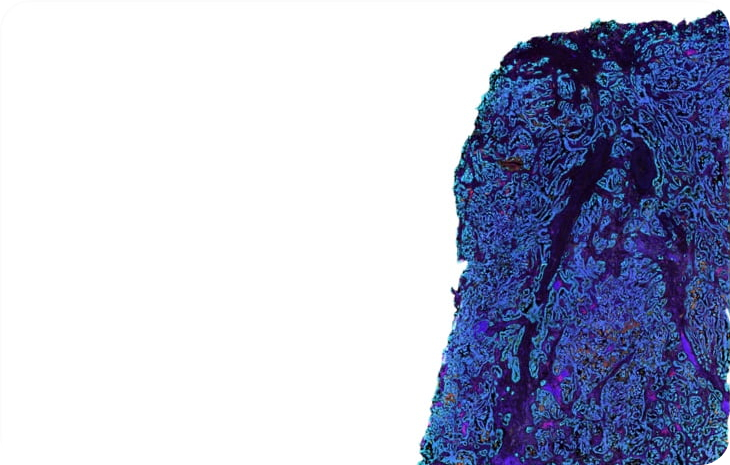